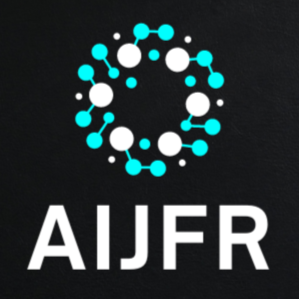
Advanced International Journal for Research
E-ISSN: 3048-7641
•
Impact Factor: 9.11
A Widely Indexed Open Access Peer Reviewed Multidisciplinary Bi-monthly Scholarly International Journal
Home
Research Paper
Submit Research Paper
Publication Guidelines
Publication Charges
Upload Documents
Track Status / Pay Fees / Download Publication Certi.
Editors & Reviewers
View All
Join as a Reviewer
Get Membership Certificate
Current Issue
Publication Archive
Conference
Publishing Conf. with AIJFR
Upcoming Conference(s) ↓
WSMCDD-2025
GSMCDD-2025
Conferences Published ↓
RBS:RH-COVID-19 (2023)
ICMRS'23
PIPRDA-2023
Contact Us
Plagiarism is checked by the leading plagiarism checker
Call for Paper
Volume 6 Issue 3
May-June 2025
Indexing Partners

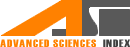
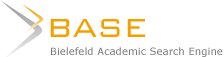

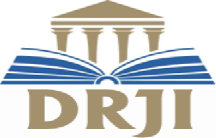

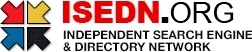

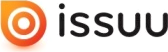

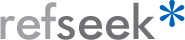
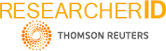
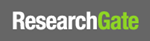
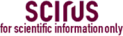
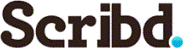

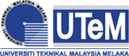

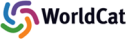
Detecting and Classifying Types of Brain Tumors Based On Location in MRI Using a Hybrid Approach
Author(s) | Anant Prakas |
---|---|
Country | India |
Abstract | Brain tumors which are said to be an abnormal state or cells that deviates from normal growth pattern in the brain, are a dangerous and life-threatening condition. Identifying these tumors and classifying them are essential for ensuring the best treatment for them. Magnetic Resonance Imaging (MRI) is widely used imaging modality for brain tumor diagnosis since, it is said to provide high-resolution images of soft tissues. However, manual interpretation is time-consuming, subjective, and prone to errors. Machine learning algorithms, particularly deep learning algorithms, have shown promise for automating this task with high accuracy. The aim of the proposed method is to identify the existence of a tumor and then is to classify them based on the major three groups i.e. meningioma, glioma, and pituitary tumor if present. This paper proposes a method which uses ENet for segmentation. Then it is followed by the process of feature extraction which is implemented using Gray Level Co-occurrence Matrix. Then the final classification is performed by ensembling pre-trained models comprising of ResNeXt-50, DenseNet-169, Inception V3 for 2- class(benign and malignant) and ShuffleNet V2, DenseNet- 169,MnasNet for three class (Glioma, meningioma and pituitary) classification. This hybrid algorithm will be evaluated using a dataset containing many brain cancer inputs that can achieve better results in both tumor cell detection and location-based types prediction accurately |
Field | Engineering |
Published In | Volume 2, Issue 2, March-April 2021 |
Published On | 2021-03-19 |
Share this

E-ISSN 3048-7641
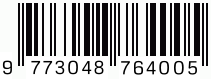
CrossRef DOI is assigned to each research paper published in our journal.
AIJFR DOI prefix is
10.36948/aijfr
All research papers published on this website are licensed under Creative Commons Attribution-ShareAlike 4.0 International License, and all rights belong to their respective authors/researchers.
